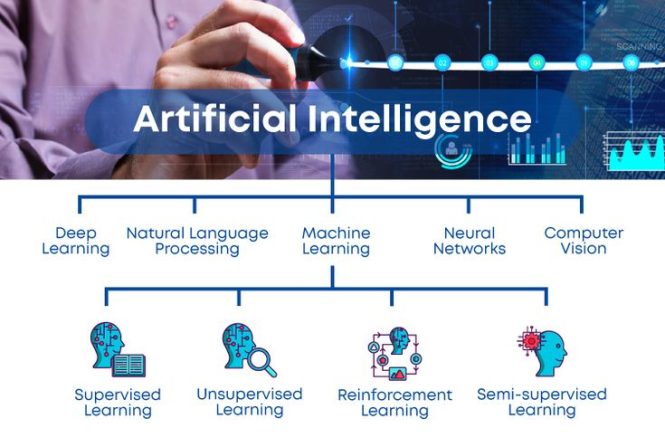
How does artificial intelligence work? This fundamental question sparks curiosity and intrigue, particularly in a world rapidly adapting to AI’s influence. AI, a branch of computer science focused on creating intelligent agents, has evolved from theoretical concepts to tangible applications impacting industries across the globe. Many individuals struggle to grasp the underlying mechanisms, leading to common misconceptions about AI’s capabilities and limitations. This comprehensive guide aims to demystify the intricate workings of AI, explaining the essential components and dispelling myths, to unlock a deeper understanding of this transformative technology. The following sections will delve into the architecture of AI systems, explore specific algorithms, and examine real-world applications, from machine learning to deep learning.
Understanding the Fundamentals of AI
Defining Artificial Intelligence
Artificial intelligence (AI) is the ability of a digital computer or computer-controlled robot to perform tasks commonly associated with intelligent beings. These tasks often include learning, problem-solving, and decision-making. AI systems are designed to mimic human cognitive functions, enabling them to analyze data, identify patterns, and make predictions. This broad definition encompasses various approaches, including rule-based systems, machine learning, and deep learning. A key goal of AI research is to create systems capable of adapting and improving their performance over time through experience.
Common Misconceptions about AI
Despite its growing presence, misconceptions about AI persist. One common misconception is that AI is solely focused on replicating human intelligence, which isn’t entirely accurate. AI systems can excel in tasks where human intelligence might be inefficient or limited. Another myth is that AI is inherently dangerous or unpredictable. While AI systems require careful design and ethical considerations, their potential benefits are vast, from enhancing efficiency in industries to resolving complex societal problems.
The Building Blocks of AI
Machine Learning: Learning from Data
Machine learning (ML) is a core component of AI, enabling systems to learn from data without explicit programming. Through algorithms, AI systems identify patterns and make predictions or decisions based on the input data. The types of learning algorithms are numerous and range from supervised learning (where the algorithm learns from labeled data) to unsupervised learning (where the algorithm finds patterns in unlabeled data) and reinforcement learning (where the algorithm learns through trial and error). Real-world applications include spam filtering, recommendation systems, and fraud detection. One notable example is the use of ML algorithms in natural language processing (NLP), enabling AI systems to understand and generate human language.
Deep Learning: Unveiling Complex Patterns
Neural Networks: Mimicking the Brain
Deep learning (DL), a subset of machine learning, leverages artificial neural networks with multiple layers. These networks mimic the structure and function of the human brain, enabling them to process complex data and extract intricate patterns. DL algorithms are particularly effective in handling large datasets and complex tasks such as image recognition and natural language processing. Consider how facial recognition systems operate—deep learning plays a central role in these systems by allowing them to identify specific facial features based on enormous amounts of training data.
Applications in Image Recognition
Deep learning algorithms are proving to be remarkably successful in image recognition tasks. For example, self-driving cars use deep learning to interpret images from cameras and sensors, enabling them to navigate roads safely. Medical imaging also benefits from these algorithms; they can assist radiologists in detecting anomalies and helping with diagnoses, offering potentially life-saving interventions.
AI in Action: Real-World Applications
AI in Healthcare
AI is revolutionizing healthcare by assisting in diagnostics, drug discovery, and personalized medicine. Algorithms can analyze medical images to detect anomalies, aiding in early disease diagnosis. Furthermore, AI systems can analyze patient data to predict disease risk and personalize treatment plans.
AI in Finance
AI is rapidly transforming the financial sector with applications ranging from fraud detection to risk assessment. Sophisticated algorithms can identify fraudulent transactions, helping financial institutions protect their clients. Moreover, AI can analyze financial data to assess risk more accurately, empowering better investment strategies.
Ethical Considerations and Future Directions
Bias and Fairness in AI
One crucial aspect of AI development is addressing potential biases in data. If training data reflects societal prejudices, the AI system may perpetuate and even amplify these biases, leading to unfair or discriminatory outcomes. Therefore, fairness and inclusivity must be prioritized in the design and development of AI systems.
Ensuring Transparency and Explainability
The increasing complexity of AI systems raises concerns about transparency and explainability. It’s essential to ensure that AI systems can be understood and audited to prevent unexpected outputs and ensure accountability.
In conclusion, Artificial Intelligence (AI) is a rapidly evolving field with significant potential to transform various industries and aspects of our lives. Understanding how AI works, from its fundamental algorithms to the complex architectures, is crucial for harnessing its power effectively. This article has explored the core concepts, common misconceptions, and practical applications. For those interested in pursuing careers in AI or simply looking to better understand the future, further exploration of specific AI branches and continued learning are recommended. Dive deeper into the fascinating world of AI by exploring related topics, and discover more resources that can support your understanding.